Journal: Hospital Practice
Publisher: Taylor and Francis
Maxwell Obubu1*, Nkata Chuku1, Alozie Ananaba1, Rodio Diallo2, Firdausi Umar Sadiq2, Emmanuel Sambo1
, Oluwatosin Kolade1, Tolu Oyenkanmi1, Kehinde Olaosebikan1, Oluwafemi Serrano3
1Health Systems Consult Limited (HSCL), Abuja, Nigeria.
2Bill and Melinda Gates Foundation, Abuja, Nigeria.
3Lagos State Health Management Agency (LASHMA), Lagos State, Nigeria.
Abstract
Background: Behind every great system is an organized team; this is especially true in the healthcare industry, where a dedicated human resources team can effectively recruit employees, train staff, and implement safety measures in the workplace. The importance of human resources in the healthcare industry cannot be overstated, with benefits ranging from providing an orderly and effectively run facility to equipping staff with the most accurate and up-to-date training. Proper human resources management is critical in providing high-quality health care. A refocus on human resources management in healthcare requires more research to develop new policies. Effective human resources management strategies are greatly needed to achieve better outcomes and access to health care worldwide.
Methods: This study leveraged NOI Polls census data on Health Facility Assessment for Lagos State. One thousand two hundred fifty-six health care facilities were assessed in Lagos State; numbers of Health workers were documented alongside their area of specialization. Also, demographic characterizations of the facilities, such as LGA, Ownership type, Facility Level Care, and Category of the facility, were also documented. Descriptive statistics alongside cross tabulation was done to present the various area of specialization of the health workers. Multiple response analysis was done to understand the distribution of human resources across the health facilities. At the same time, Chi-square and correlation tests were conducted to test the independence of various categories recorded while understanding the relationships among selected specialties.
Results: The study revealed that Nurses were the most common health specialist in the Lagos State health facilities. At the same time, Gynecologists and General surgeons are the two medical specialists mostly common in health facilities. Midwives are the second most common health specialist working full time, while Generalist medical doctors make up the top three health specialists working full time. Nurses and Midwives had the highest number in Lagos State, while Pulmonologists were currently the lowest human resource available in Lagos State health care system. It was also noted that health facility distribution across Lagos’s urban and rural areas was even. In contrast, distribution based on other factors such as ownership type, Facility level of care, and facility category was slightly skewed.
Conclusion: The distribution of health workers in health facility across LGA in Lagos State depend on Ownership type, Facility level of care, and category of the facility.
1. Introduction
Every health system revolves around health workers [1] . In order to provide health services to those in need, their knowledge, skills, and motivation are essential. Many nations are struggling to meet enormous human resource needs for health policy issues like addressing surpluses or shortages and enhancing health workers’ knowledge, geographic distribution, and performance. The World Bank’s agenda for strengthening health systems still depends on improving the health workforce [1] .
A nation’s capacity to achieve its health goals is largely determined by the knowledge, skills, training, motivation, and deployment of personnel in charge of planning and delivering healthcare. Several studies show a direct and positive link between the number of health workers and population health outcomes [1] [2] . Many countries lack the human resources needed to deliver essential health interventions for several reasons, including limited production capacity, migration of health workers within and across countries, poor skills, and demographic imbalances. Formulating national policies and plans in pursuit of human resources for health development objectives requires good information and evidence. Against the backdrop of increasing demand for information, building knowledge and databases on the health workforce requires coordination across sectors.
The World Health Organization (WHO) is engaging countries and partners to strengthen the global evidence base on the health workforce—which includes gaining consensus on a core set of indicators and minimum data set for monitoring health workers’ stock, distribution, and production. The term “health workforce” refers to all individuals engaged in activities with the primary goal of promoting health [3] . The human resources for health (HRH) include clinical staff such as physicians, nurses, midwives, pharmacists, and dentists. It also includes managerial and support staff such as those who do not deliver services directly but are important to the performance of health systems. There are currently no thorough and reliable methodologies for determining whether a given population’s healthcare needs can be met by a given health workforce [3] . According to WHO estimates, nations with fewer than 23 medical professionals—doctors, nurses, and midwives—per 10,000 people typically fall short of meeting the Millennium Development Goals’ targets for coverage rates for a number of primary healthcare interventions [3] . Several factors contribute to perceived shortages of health professionals, including losses brought on by deaths, retirement, career changes, or out-migration, as well as insufficient numbers and a mismatch in the skills of those being trained. At all levels, both resource-poor and wealthier countries have widely acknowledged the need for comprehensive, trustworthy, and timely information on HRH, including numbers, demographics, skills, services being provided, and factors influencing recruitment and retention. Given the global initiative to increase health worker education and training in 57 countries, mostly in sub-Saharan Africa, which has been identified as having a critical shortage of highly skilled health professionals, this HRH need has become even more urgent [3] .
A robust human resources component in a health information system can help build the evidence base needed to plan for the availability and accessibility of required health workers in the right place and time and in the desired quality [4] . The Planning requires insight into the number of active health workers in the health sector, distribution, and characteristics. It also requires knowledge of the numbers and characteristics of those being trained and added to the human resources pool, those leaving the active workforce, and the reasons for leaving [4] [5] . A robust human resources information system (HRIS) can also guide decision-making to ensure the cultural appropriateness of the health system and encourage the utilization of services among underserved regions. Access to female providers, for example, is an essential determinant of women’s health service utilization patterns in some contexts [6] . Promoting the collection and use of sex-disaggregated data in all human resource assessments should be part of a strategy for ensuring the male-female balance of the health workforce. A timely, dependable, and relevant HRIS is required to aid in developing, monitoring, and evaluating health workforce plans, strategies, and policies at the subnational, national, and international levels. Unfortunately, for the majority of countries, there is still a significant gap between the demand for data and the availability and utility of the information needed to support decision-making [6] . The study, therefore, looks into the distribution of health facilities and human resources in Lagos State.
2.1. Data and Sampling Methods
The study leveraged primary data collected on health facilities assessment in Lagos State by NOI Polls. NOI Polls adopted a quantitative research methodology for the health facility assessment. At the same time, HSCL developed a list of health facilities using information from the State Ministry of Health (SMOH). The list served as a sample frame for health facilities in Lagos State. The sampling frame consisted of 2398 health facilities, and a census approach was adopted. The data collection method used was Computer Assisted Telephone Interview (CATI). Health facilities’ target respondents (chief medical directors, medical directors, facility administrators) were interviewed over the telephone using a Questionnaire Processing Software for Market Research (QPSMR). The telephone interview call protocol specifies that each health facility in the sample frame is attempted six times for an interview before the health facility falls into the category of an unsuccessful call. NOI Polls engaged vital stakeholders to refine the technical assistance plan for the health facility assessment. These stakeholders included the State Ministry of Health (SMOH), Lagos State Health Management Agency (LASHMA), the Health Facility Monitoring and Accreditation Agency (HEFAMAA), Association of General and Private Medical Practitioners of Nigeria (AGPMPN), and other relevant professional bodies. The final health facility assessment dataset contains information on Facility Ownership, Facility level of Care, Accrediting body, Human Resources for Health, Basic Medical & Infection Prevention Equipment, Infrastructure, Available Services, Health Insurance Coverage, Medicines & Commodities, Financial Management Systems, Clinical Governance, and Covid-19 Response.
2.2. Statistical Method
Based on the nature of the study and the objective, the following relevant inferential statistics were used.
2.3. Chi-Square Tests
The Chi-Square Test of Independence determines whether there is an association between categorical variables (i.e., whether the variables are independent or related). It is a nonparametric test. The null hypothesis (H0) and alternative hypothesis (H1) of the test is stated below:
Null hypothesis: “[Variable 1] is independent of [Variable 2].”
Alternative hypothesis: “[Variable 1] is not independent of [Variable 2].”
(1)
Where
is the observed cell count in the ith row and jth column of the table;
is the expected cell count in the ith row and jth column of the table, computed as
To make a decision, we compute and compare the calculated X2 value with the critical value. The critical X2 value is obtained from the X2 distribution table with degrees of freedom and chosen confidence level. If the calculated X2 value is greater than the critical X2 value, we reject the null hypothesis.
2.4. Independent Sample t-Tests
We use Independent Samples t-test to determine if two groups are significantly different from each other on the variable of interest. The variable of interest should be continuous, be normally distributed, and have a similar spread between the two groups. The independent sample t-test is an inferential statistical hypothesis test that uses samples to draw valuable conclusions about populations.
Independent sample t-tests have the following hypothesis:
· H0: The means for the two populations are equal;
· H1: The means for the two populations are not equal.
The null hypothesis (H0) is rejected if the p-value is lower than the significance level (e.g., 0.05), implying that the difference between the two means is statistically significant and that the sample provides strong evidence to conclude that the two population means are not equal. Assuming equal variance is observed, the independent t-test statistic is given by the formula below:
(2)
Where
= Mean of the first sample;
= Mean of the second sample;
= Sample size (i.e., number of observations) of the first sample;
= Sample size (i.e., number of observations) of the second sample;
= Standard deviation of the first sample;
= Standard deviation of the second sample.
2.5. One Way Analysis of Variance
The one-way analysis of variance is a parametric test that compares the means of two or more independent groups to determine whether there is statistical evidence that the associated population means are significantly different.
The null and alternative hypotheses can be expressed as:
H0:
H1: At least one different
where is the population mean of the ith group ( ).
The F statistic is computed as follows:
(3)
3. Results and Discussion
Table 1 shows that Alimosho local government area (LGA) has the highest number of health facilities in Lagos State (221; 17.6%); this is closely followed by Surelere LGA with 114 (9.1%) health facilities, then Ikorodu LGA with 100 (8.0%) health facilities. It also reveals the number of primary and secondary healthcare facilities considered for this study.
Nurses are the most common health workers available in health facilities across the state, with 75.8% of health facilities having at least one nurse. Altogether, nurses (75.8%), midwives (57.8%), and generalist medical doctors (49.0%) make up the top three most common health workers in health facilities (Table 2).
LGA | Number of Health Facilities | % | Level of Care | Number of Health Facilities | % | Category | Number of Health Facilities | % | ||
Alimosho | 221 | 17.6 | Tertiary Facility | 1 | 0.1 | Private Hospital | 489 | 38.9 | ||
Surulere | 114 | 9.1 | SHC Facility | 309 | 24.6 | (Medical) Clinic (PHC) | 245 | 19.5 | ||
Ikorodu | 100 | 8.0 | PHC Facility | 946 | 75.3 | Laboratory | 82 | 6.5 | ||
Eti-Osa | 93 | 7.4 | Tertiary Facility | Maternity Home | 79 | 6.3 | ||||
Kosofe | 80 | 6.4 | Government/Public | 1 | 100.0 | Convalescent/Nursing Home | 76 | 6.1 | ||
Ojo | 75 | 6.0 | SHC Facility | Eye Clinic | 61 | 4.9 | ||||
Ikeja | 73 | 5.8 | Government/Public | 6 | 1.9 | Diagnostic (Lab, Scan, ECG, MRI+CT Scan) | 55 | 4.4 | ||
Oshodi-Isolo | 70 | 5.6 | Private-For-Profit | 298 | 96.4 | Government Hospital/Clinic | 53 | 4.2 | ||
Ifako-Ijaye | 56 | 4.5 | Others (NGOs, Mission/Faith-Based) | 5 | 1.6 | Dental Clinic | 51 | 4.1 | ||
Ajeromi-Ifelodun | 52 | 4.1 | PHC Facility | Specialist Clinic/Hospital | 35 | 2.8 | ||||
Amuwo-Odofin | 51 | 4.1 | Government/Public | 187 | 19.8 | Specialist Clinic | 25 | 2.0 | ||
Mushin | 48 | 3.8 | Private-For-Profit | 743 | 78.5 | Industrial Facility | 3 | 0.2 | ||
Badagry | 37 | 2.9 | Others (NGOs, Mission/Faith-Based) | 16 | 1.7 | Physio | 2 | 0.2 | ||
Agege | 36 | 2.9 | ` | |||||||
Shomolu | 36 | 2.9 | ||||||||
Ibeju/Lekki | 31 | 2.5 | ||||||||
Lagos Island | 28 | 2.2 | ||||||||
Lagos Mainland | 27 | 2.1 | ||||||||
Apapa | 16 | 1.3 | ||||||||
Epe | 12 | 1.0 | ||||||||
Total | 1,256 | 100 |
Table 1. Distribution of health facilities assessed by LGA, level of care, and category.
Total | Facility Ownership Type | Facility's Level of Care | ||||
Government/ Public | Private-For-Profit | Others (NGOs, Mission/Faith-Based) | SHC Facility | PHC Facility | ||
Nurses | 75.8 | 87.1 | 73.3 | 90.5 | 35.9 | 88.6 |
Midwives | 57.8 | 63.4 | 56.5 | 71.4 | 21.1 | 69.7 |
Generalist (non-specialist) medical doctors | 49.0 | 36.6 | 51.1 | 57.1 | 21.1 | 58.0 |
Specialist medical doctors | 46.1 | 5.2 | 53.5 | 57.1 | 57.2 | 42.4 |
Nursing assistant or technician | 40.3 | 8.2 | 46.2 | 47.6 | 17.8 | 47.6 |
Community Health Workers (CHEW) | 35.6 | 78.4 | 27.6 | 33.3 | 10.9 | 43.6 |
Laboratory technicians (medical and pathology) | 33.9 | 45.9 | 31.2 | 57.1 | 40.1 | 32.0 |
Laboratory scientists | 31.3 | 16.0 | 33.8 | 47.6 | 52.6 | 24.4 |
Pharmacy technicians/scientists | 28.1 | 61.9 | 21.5 | 38.1 | 11.8 | 33.3 |
Medical records officers | 27.3 | 34.5 | 26.1 | 19.0 | 13.5 | 31.7 |
Community Health Workers (CHO) | 17.6 | 55.2 | 10.7 | 9.5 | 3.6 | 22.1 |
Pharmacists | 11.8 | 21.6 | 9.7 | 28.6 | 6.3 | 13.7 |
Physiotherapists | 9.4 | 1.0 | 10.9 | 14.3 | 7.6 | 10.1 |
Community Health Workers (JCHEW) | 8.6 | 14.4 | 7.7 | 0.0 | 3.0 | 10.5 |
Radiographers | 8.3 | 0.5 | 9.7 | 14.3 | 15.5 | 6.0 |
Others | 9.4 | 6.7 | 10.0 | 9.5 | 14.1 | 7.9 |
Table 2. Percentage of health facilities having at least 1 health specialist.
Gynecologists an3 general surgeons are the two most common medical specialists in health facilities, with a percentage of 24.1% and 21.1%, respectively. The top 3 specialist medical doctors available across health facilities in the state were found to be obstetricians/gynecologists (24.1%), general surgeons (21.1%), and pediatricians (15%). Additionally, disaggregation across health facility types revealed that government-owned health facilities had a limited number of these top 3 specialist medical doctors compared to private for-profit and NGO/Mission/FBO-owned health facilities (Table 3).
Table 4 gives the case summary of the multiple response frequencies of available human resources in Lagos State. There are 1256 cases in the data collected on human resource availability in Lagos State, Nigeria, six of which are considered
Total | Facility Ownership Type | Facility's Level of Care | ||||
Government/ Public | Private-For-Profit | Others (NGOs, Mission/Faith-Based) | SHC Facility | PHC Facility | ||
Obstetrician/Gynecologist | 24.1 | 2.1 | 28.0 | 33.3 | 15.1 | 27.0 |
General Surgeon | 21.1 | 2.1 | 24.5 | 28.6 | 13.5 | 23.6 |
Pediatrician | 15.0 | 0.5 | 17.7 | 14.3 | 13.8 | 15.3 |
Anesthetist | 11.7 | 0.5 | 13.8 | 9.5 | 8.2 | 12.8 |
Orthopedic Surgeon | 11.3 | 1.0 | 13.2 | 9.5 | 10.2 | 11.6 |
Optometrist | 10.7 | 0.5 | 12.6 | 14.3 | 18.1 | 8.3 |
Cardiologist | 10.6 | 0.5 | 12.6 | 4.8 | 11.8 | 10.2 |
Dentist | 9.1 | 0.5 | 10.8 | 4.8 | 15.1 | 7.2 |
Urologist | 7.8 | 1.0 | 9.0 | 9.5 | 6.6 | 8.1 |
Psychiatrist | 6.3 | 0.5 | 7.3 | 9.5 | 5.9 | 6.5 |
Radiologist | 6.3 | 0.5 | 7.5 | 0.0 | 7.2 | 6.0 |
Ophthalmologist | 5.9 | 1.0 | 7.0 | 0.0 | 9.2 | 4.8 |
Hematologist//Oncologist | 5.4 | 0.0 | 6.3 | 9.5 | 3.9 | 5.8 |
Endocrinologist | 5.3 | 0.5 | 6.3 | 0.0 | 6.9 | 4.8 |
Neurosurgeon | 4.2 | 0.0 | 5.0 | 4.8 | 4.3 | 4.2 |
Dermatologist | 3.6 | 0.0 | 4.3 | 4.8 | 3.9 | 3.5 |
Intensivist | 2.6 | 0.0 | 3.1 | 4.8 | 3.3 | 2.4 |
Pulmonologist | 2.0 | 0.0 | 2.4 | 0.0 | 2.0 | 2.0 |
Others | 2.9 | 0.0 | 3.5 | 0.0 | 2.0 | 3.2 |
Table 3. Percentage of health facilities having at least 1 specialist medical doctor.
Cases | ||||||
Valid | Missing | Total | ||||
N | Percent | N | Percent | N | Percent | |
$Healthworkersa | 1250 | 99.5% | 6 | 0.5% | 1256 | 100.0% |
Table 4. Case summary of the multiple response frequencies of available human resources in Lagos State.
aDichotomy group tabulated at value 1.
“missing”. These are facilities that do not have any of the listed healthcare providers. The frequency table for multiple responses set with available specialty areas in healthcare delivery coded as dichotomies provided similar information to the frequency tables for individual variables; however, the results were more compact, and some extra information was also recorded. N is the number of specialized human resources available in health facilities. Nurses and Midwives had the highest number in Lagos State, with 11.5% and 8.8%, respectively, among 35 areas of specialty. Pulmonologists were currently the lowest human resource in Lagos State health care system.
We use the Student’s Independent samples t-test (Table 5) to test the null hypothesis that two groups, i.e., Urban and Rural (Locality), have the same Mean. A high p-value suggests that the null hypothesis is true, and therefore the group means are not different. The result from the analysis is reported above for the Student’s t, Wench’s t, and Mann-Whitney U test (P < 0.339, 0.318, and 0.657, respectively). The result is good in that it depicts that rural communities are not left out in the distribution of healthcare facilities in Lagos State. From a Bayesian perspective, the null hypothesis states that there is no mean (average) difference between the two groups, i.e., Urban and Rural (The alternative Hypothesis, on the other hand, is that there is a mean difference between the two groups) [7] [8] . Bayes Factor10 (BF10) equals 0.118, the result further implies that the observed data support 0.118 times more for the alternative hypothesis (i.e., there is a mean difference of health workers between the two groups of the Locality variable, i.e., Urban and Rural) than for the null Hypothesis (A mean difference of health workers between the two groups of the Locality variable does not exist). The error % (error percentage) tells whether the numerical results are robust. According to the error % column, the error percentage is 6.31e−6, which equals 6.31 × 10−6. Since the error percentage is low enough, we can say that the numerical results are robust. The Student’s independent t-test assumes that the data from each group are from a normal distribution and that the variances of these groups are equal. If unwilling to assume the groups have equal variances, Welch’s t-test can be used in its place [9] . If one is unwilling to assume the data from each group are from a normal distribution, the nonparametric Mann-Whitney U test can be used instead (However, note that the Mann-Whitney U test has a slightly different null hypothesis; that the distributions of each group are equal) [7] . This informed on why we carried out all three tests. As shown in Table 6, the normality test carried out using the Shapiro-Wilk test also confirmed the violation of the normality assumption. So, since Levene’s test gave a p-value greater than 0.05, our decision is based on Student’s t-test because it assumes that variances are equal. Figure 1 shows the mean and
Independent Samples T-Test | |||||||||||||
Statistic |
±% | df | P | Mean difference | SE difference | 95% Confidence Interval | Effect Size | 95% Confidence Interval | |||||
Lower | Upper | Lower |
Upper |
||||||||||
No. of Health workers |
Student's t |
-0.957 |
6.31e-6 |
1254 | 0.339 | -0.354 | 0.370 | -1.08 | 0.372 | Cohen's d | -0.0643 | -0.196 | 0.0676 |
Bayes factor10 | 0.118 | ||||||||||||
Welch's t | -0.999 | 501 | 0.318 | -0.354 | 0.355 | -1.05 | 0.342 | Cohen's d | -0.0657 | ||||
Mann-Whitney U
|
136664 | 0.657 | -4.60e-5 | -1.000 | 2.59e-5 | Rank biserial correlation | 0.0172 |
Table 5. Human resources in Lagos State health facilities are grouped by locality using independent t-test.
Homogeneity of Variances Test (Levene's) |
||||||||
|
F | df | Df2 |
p |
||||
No. of Health Workers | 2.29 | 1 | 1254 | 0.131 | ||||
Note. A low p-value suggests a violation of the assumption of equal variance | ||||||||
Normality Test (Shapiro-Wilk) |
||||||||
W |
p |
|||||||
No. of Health Workers | 0.820 | <.001 | ||||||
Note. A low p-value suggests a violation of the assumption of Normality | ||||||||
Group Descriptives |
||||||||
Group |
N | Mean | Median | SD |
SE |
|||
No. of Health Workers | Rural | 287 | 6.26 | 4.00 | 5.17 | 0.305 | ||
Urban | 969 | 6.61 | 5.00 | 5.60 | 0.180 |
Table 6. Test for violation of assumption guiding the use of t-test
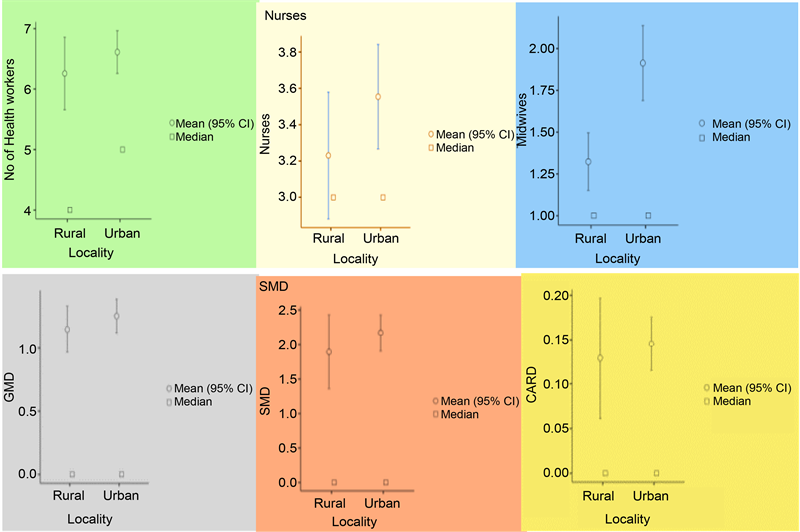
Figure 1. Mean and median distribution of specialized areas in Lagos State human resources for health.
median distribution of specialized areas in Lagos State human resources for health.
The Analysis of Variance (ANOVA) in Table 7 was used to explore the relationship between the total number of human resources in each facility and their
One Way Anova of Available Human Resources in Health using LGA |
|||||
F |
df1 | df2 |
P |
||
No. of Health Workers | Welch's | 2.56 | 19 | 278 | <.001 |
Fisher's | 2.23 | 19 | 1236 | 0.002 | |
One Way Anova of Available Human Resources in Health using Ownership Type |
|||||
F |
df1 | df2 |
p |
||
No. of Health Workers | Welch's | 10.9 | 2 | 53.0 | <.001 |
Fisher's | 4.46 | 2 | 1253 | 0.012 | |
One Way Anova of Available Human Resources in Health using Category of Facilities |
|||||
F |
df1 | df2 |
p |
||
No. of Health Workers | Welch's | 66.9 | 12 | 34.9 | <.001 |
Fisher's | 32.2 | 12 | 124.3 | <.001 |
Table 7. Analysis of Variance (ANOVA) of available human resources in health using LGA, Ownership type, and category of facilities.
categorical explanatory variables, namely LGA, Ownership Type, and Facility Category. This “One-Way ANOVA” is a simplified version of the “normal” ANOVA, allowing only a single explanatory factor but also providing Welch’s ANOVA. Welch’s ANOVA has the advantage that it need not assume that the variances of all groups are equal [10] [11] . This method specifies multiple dependent variables for convenience, resulting in multiple independent tests. The null hypothesis was rejected in all three categories stating that the number of human resources available differs across LGA, ownership type, and facility category. This result negated the comparison done with locality under the t-test in Table 5, that the number of health workers is equal across rural and urban communities.
Correlation matrices can examine linear relationships between two or more continuous variables. A Pearson’s r value for each pair of variables shows the magnitude and direction of the relationship between those two variables. A positive value denotes a positive relationship (higher values of one variable predict higher values of the other variable). A negative Pearson’s r denotes a negative relationship (higher values of one variable predict lower values of the other variable, and vice-versa). A zero-correlation value means no relationship exists when the variables [12] [13] . More formally, it is possible to test the null hypothesis that the correlation is zero and calculate a p-value. If the p-value is low, it suggests the correlation coefficient is not zero and there is a linear (or more complex) relationship between the two variables. This was done using the number of essential specialists expected to be attached to each health facility. Figure 2 shows the correlation coefficients and the relationship’s direction among the selected specialties. The plot revealed that most of the GMD had BLS/ALS certifications. Increased relationships were also noticed between the number of Nurses and Midwives available in all the health facilities enumerated in Lagos State.
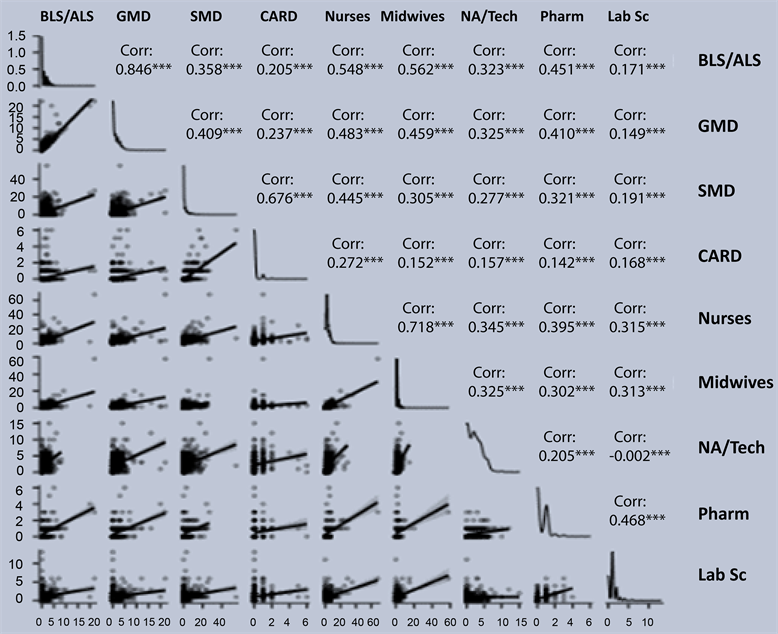
Figure 2. Correlation plot of available specialized areas within the health facilities in Lagos State.
The χ2 test of association (not to be confused with the χ2 goodness of fit) is used to test whether two categorical variables are independent or associated [14] [15] . If the p-value is low, it suggests that the variables are not independent and that there is a relationship between the two variables. This study used the Chi-square test to determine whether the number of health facilities in specified groups significantly differs. So, the chi-square test was used to answer the question: Does the number of health facilities in the locality (urban/rural) differ significantly in ownership type, level of care, and category of the facility? In the results displayed in Tables 8-10, the p-value associated with the interaction of locality, ownership type, facility level of care, and category of the facility was less than 0.05, i.e., p < 0.05. This result means that the distribution of health facilities [dt_gap height="20" /]
X2 Tests | |||||||
Locality |
Value | df |
P |
||||
Rural | X2 | 126.6 | 38 | <.001 | |||
N | 287 | ||||||
Urban |
X2 |
97.8 | 38 | <.001 |
Nominal |
||
N | 969 | Locality | Value | ||||
Total |
X2 |
163.8 | 38 | <.001 | Rural | Contingency coefficient | 0.553 |
N | 1256 | Urban | Contingency coefficient | 0.303 | |||
Total | Contingency coefficient | 0.340 |
Table 8. Chi-Square analysis based on the distribution of human resources across locality and facility ownership type.
X2 Tests | |||||||
Locality |
|
Value | df |
P |
|||
Rural | X2 | NaN | 38 |
NaN |
|||
N |
287 |
||||||
Urban | X2 | 50.5 | 38 |
0.084 |
Nominal |
||
N |
969 |
Locality |
Value |
||||
Total | X2 |
76.7 |
38 |
<.001 |
Rural |
Contingency coefficient |
NaN |
N |
1256 |
Urban |
Contingency coefficient |
0.223 |
|||
Total |
Contingency coefficient |
0.240 |
Table 9. Chi-Square analysis based on the distribution of human resources across locality and facility level care.
X2 Tests | |||||||
Locality | Value | df | P | ||||
Rural |
X2 | NaN | 228 |
NaN |
|||
N |
287 |
||||||
Urban | X2 | 410 | 228 |
0.084 |
Nominal |
||
N |
969 |
Locality |
Value |
||||
Total | X2 | 497 | 228 |
<.001 |
Rural |
Contingency coefficient |
NaN |
N |
1256 |
Urban |
Contingency coefficient |
0.545 |
|||
Total |
Contingency coefficient |
0532 |
Table 10. Chi-Square analysis based on the distribution of human resources across locality and facility category.
across the locality does depend on facility ownership type, facility level of care, and category of the facility in Lagos State.
4. Conclusion
This paper studied the distribution of human resources for health in 1256 health facilities in Lagos State. Nurses are the most common health specialist in health facilities, Gynecologists and general surgeons are the two medical specialists mostly common in health facilities, and Midwives are the second most common health specialist working full time. Generalist medical doctors make up the top three health specialists working full-time. Nurses and Midwives had the highest number in Lagos State, while Pulmonologists were currently the lowest human resource available in the Lagos State health care system. It was also noted that health facility distribution across Lagos’s urban and rural areas was even. In contrast, distribution across other factors such as ownership type, facility level of care, and facility category was slightly skewed. Hence, the distribution of health workers in health facilities across the different LGAs depends on ownership type, facility level of care, and facility category.
Authors Contribution
All authors contributed equally to the conception and design of the study
Funding
This research is part of the work supported by the Bill and Melinda Gates Foundation (BMGF) through the Nigerian-led Strategic Purchasing for Family Planning grant [grant number: INV-007359] assigned to HSCL.
Conflicts of Interest
The authors declare that they have no financial or personal relationships that may have inappropriately influenced them in writing this article.
References
[1] | World Bank (2013) Human Resource for Health. The World Bank. |
[2] | Anand, S. and Barnighausen, T. (2007) Health Workers and Vaccination Coverage in Developing Countries: An Econometric Analysis. The Lancet, 369, 1277-1285. https://doi.org/10.1016/S0140-6736(07)60599-6 |
[3] | World Health Organization (2006) Working Together for Health. The World Health Report, WHO, Geneva. |
[4] | Diallo, K., Zurn, P., Gupta, N., et al. (2013) Monitoring and Evaluation of Human Resources for Health: An International Perspective. Human Resource for Health, 1, Article No. 3. https://doi.org/10.1186/1478-4491-1-3 |
[5] | World Health Organization, World Bank and United States Agency for International Development (2009) Handbook on Monitoring and Evaluation of Human Resources for Health. Geneva. |
[6] | Sen, G., Ostlin, P. and George, A. (2007) Gender Inequity in Health: Why It Exists and How We Can Change It. WHO Commission on the Social Determinants of Health. |
[7] | Morey, R.D. and Rouder, J.N. (2022) BayesFactor: Computation of Bayes Factors for Common Designs. https://cran.r-project.org/package=BayesFactor |
[8] | Rouder, J.N, Speckman, P.L., et al. (2009) Bayesian t Tests for Accepting and Rejecting the Null Hypothesis. Psychonomic Bulletin & Review, 16, 225-237. https://doi.org/10.3758/PBR.16.2.225 |
[9] | R Core Team (2018) R: A Language and Environment for Statistical Computing. https://cran.r-project.org/ |
[10] | Meyer, D., Zeileis, A., et al. (2009) VCD: Visualizing Categorical Data. |
[11] | Lenth, R.V. (2021) Emmeans: Estimated Marginal Means, Aka Least-Squares Means. https://cran.r-project.org/package=emmeans |
[12] | Kerby, D.S. (2014) The Simple Difference Formula: An Approach to Teaching Nonparametric Correlation. Comprehensive Psychology, 3, 2165-2228. https://doi.org/10.2466/11.IT.3.1 |
[13] | Fox, J., Weisberg, S. and Price, B. (2022) Car: Companion to Applied Regression. https://cran.r-project.org/package=car |
[14] | Jamovi (2021) The Jamovi Project (Version 1.8). Computer Software. https://www.jamovi.org/ |
[15] | Speybroec, N., Kinfu, Y., et al. (2006) Reassessing the Relationship between Human Resources for Health, Intervention Coverage and Health Outcomes. World Health Organization, Geneva. |